本文已被:浏览 1190次 下载 938次 |
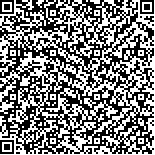 码上扫一扫! |
育肥猪生长过程中脸部变化对识别模型准确率的影响 |
张建龙1,2,周康1,2,庄晏榕1,2,滕光辉1,2,3*
|
|
(1.中国农业大学 水利与土木工程学院, 北京 100083;2.农业农村部设施农业工程重点实验室, 北京 100083;3.北京市畜禽健康养殖环境工程技术研究中心, 北京 100083) |
|
摘要: |
为探究猪脸识别模型对育肥猪猪脸生长变化的识别效果,采用深度卷积网络,对大白育肥猪生长过程中脸部变化与识别模型准确率之间的关系进行研究。结果表明:1)在预训练的DenseNet201、MobileNetV3_small、SeNet154和Xception 4 种模型中,SeNet154模型猪脸识别效果最好,对验证集的识别准确率可达98.80%,选其为猪脸识别模型;2)分别用试验期间第1~2、1~3、1~4和1~5天采集的猪脸图像数据训练该模型,并分别使用第3~8、4~9、5~10和6~11天的数据逐天测试模型的猪脸识别效果发现,使用第1~4与1~5天数据训练的模型猪脸识别效果相当且优于使用第1~2与1~3天数据训练的模型。使用第1~4天数据训练的模型对第5天数据的识别准确率最高,为96.74%,其后5天的识别准确率逐天下降,到第10天为84.17%。分析认为,造成识别准确率下降的原因是猪脸生长、光照变化以及污渍附着。3)使用第(n-4)~(n-1)天(5≤n≤10)的数据重新训练动态模型后,对第n天猪脸数据的识别准确率均在95.82%以上。深度卷积网络模型在猪脸识别方面有较高的识别准确率,可以用于育肥猪的脸部识别;建议在育肥猪的猪脸识别系统中每天都使用至少前4天,且≥10 800组图像数据重新更新猪脸识别模型。 |
关键词: 育肥猪 猪脸识别 深度学习 卷积神经网络 |
DOI:10.11841/j.issn.1007-4333.2021.06.18 |
投稿时间:2020-10-11 |
基金项目:“十三五”国家重点研发计划(2016YFD0700204) |
|
Effect of facial changes on the accuracy of the recognition model during the growth of finishing pigs |
ZHANG Jianlong1,2,ZHOU Kang1,2,ZHUANG Yanrong1,2,TENG Guanghui1,2,3*
|
(1.College of Water Resources and Civil Engineering, China Agricultural University, Beijing 100083, China;2.Key Laboratory of Agricultural Engineering in Structure and Environment of Ministry of Agriculture and Rural Affairs, Beijing 100083, China;3.Beijing Engineering Research Center on Animal Healthy Environment, Beijing 100083, China) |
Abstract: |
In order to study the effects of the pig face recognition model on the face change recognition during finishing pigs' growth, this study used deep convolutional networks to investigate the relationship between facial changes during Large White finishing pigs' growth and the accuracy of recognition models. The results showed that: 1)Comparision of the results of four pre-trained pigface recognition models(DenseNet201, MobileNetV3_small, SeNet154 and Xception)in experiments discovered that the pig face recognition accuracy of SeNet154(98. 80% in validation set)was the highest, and was then selected as pig face recognition model in this study. 2)The model was trained using the data of first few days after experiment operated, and then tested by using the daily data of the next 6 days, respectively. It was found that the recognition accuracy of the model trained with the data of the first 4 days after the experiment operated was equivalent with that the model trained with the data of first 5 days, and was higher than the model trained with the data of the first 2 and 3 days. The recognition accuracies of the model trained with the data of the first 4 days were gradually decreased to test the daily data of next 6 days, and the recognition accuracies were 96. 74% and 84. 17% to test the data of the 5th day and 10th day, respectively. The reasons for the recognition accuracies decreasing were due to the growth of pig faces, illumination changes inside the pig house, and adhesion of stains. 3)The recognition accuracies of using the dynamic model updated daily with the data of 4 days before the tested day to recognize pig face data of the tested day were all above 95. 82%. Therefore, the deep convolutional network model has high recognition accuracy on pig face, and it can be used for finishing pig's facial recognition. Due to the finishing pigs grow fast, it is recommended that the pig face recognition model should be updated every day using the data of previous 4 days or more and the face images without less than 10 800 sets. |
Key words: finishing pigs pig face recognition deep learning convolutional neural network |