本文已被:浏览 801次 下载 523次 |
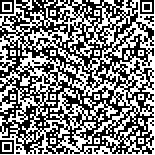 码上扫一扫! |
基于K-means-BP神经网络的舍饲环境母羊产前运动行为分类识别 |
刘艳秋1,2,宣传忠1*,武佩1,张丽娜3,张永安2,李美安2
|
|
(1.内蒙古农业大学 机电工程学院, 呼和浩特 010018;2.内蒙古农业大学 计算机与信息工程学院, 呼和浩特 010018;3.内蒙古师范大学 物理与电子信息学院, 呼和浩特 010022) |
|
摘要: |
为了探讨舍饲圈养方式对怀孕母羊生理及心理健康产生的影响,设计以三轴加速度传感器MPU6050为核心的母羊产前运动行为监测装置,并对37只成年小尾寒羊母羊产前的运动行为(站立、行走、趴卧及刨地)进行分类识别。针对母羊趴卧和站立2种静态行为识别难度大的问题,提出采用K-means聚类算法对趴卧行为进行识别。在此识别结果的基础上,通过MATLAB软件对其他3种行为数据进行校正、滤波、加窗分段处理,提取母羊产前运动行为数据的19维特征,通过主成分分析降维方法获取累积贡献率达到95%的6个分量作为新的特征集用于行为识别。利用BP神经网络算法对其他3种运动行为进行分类识别。结果表明:所设计的母羊产前运动行为识别算法对母羊产前趴卧行为的识别率达到99.2%,对站立、行走及刨地行为的识别率分别为85.7%、88.9%、80.0%,能够满足对母羊行为识别的要求;经PCA降维后算法的识别率提高了9.9%,识别效果得到了改善。 |
关键词: 母羊 舍饲圈养 产前行为 聚类算法 神经网络 行为识别 |
DOI:10.11841/j.issn.1007-4333.2021.03.10 |
投稿时间:2020-06-18 |
基金项目:内蒙古自然科学基金项目(2020BS03038,2020BS06003);国家自然科学基金项目(31860666,31960494);内蒙古农业大学基础学科科研启动基金(JC2018006);内蒙古农业大学高层次人才引进科研项目(NDYB2017-31) |
|
Classification and recognition on movement behaviors of ewes in house feeding environment based on K-means and BP neural network |
LIU Yanqiu1,2,XUAN Chuanzhong1*,WU Pei1,ZHANG Lina3,ZHANG Yongan2,LI Meian2
|
(1.College of Mechanical and Electrical Engineering, Inner Mongolia Agricultural University, Hohhot 010018, China;2.College of Computer and Information Engineering, Inner Mongolia Normal University, Hohhot 010018, China;3.College of Physics and Electronic Information Science, Inner Mongolia Normal University, Hohhot 010022, China) |
Abstract: |
In order to investigate the effects of house feeding on the physiological and mental health of pregnant ewes, a monitoring device of ewe prenatal movement behavior based on three-axis acceleration sensor MPU6050 was designed, and 37 adult Small-Tail Han ewes' prenatal movement behaviors(standing, walking, lying and digging)were classified and identified. To solve the difficulty in identifying two static behaviors of ewe' lying and standing behavior, the K-means clustering algorithm was adopted to identify the ewes' lying behavior. On the basis of the recognition results, the other three kinds of behavior data were corrected, filtered and windowed by MATLAB software, and 19 dimensional feature values of ewes' movement data were extracted. The principal component analysis method was applied to obtain the six components whose cumulative contribution rate was up to 95%. BP neural network algorithm was then used to identify the other three kinds of movement behaviors(standing, walking and digging). The results showed that the designed ewes' prenatal movement behavior recognition algorithm could recognize ewes' prenatal lying behavior with the recognition rate of 99. 2%. The recognition rates of standing, walking and digging were 85. 7%, 88. 9%, and 80. 0%, respectively, which could satisfy the requirements of ewes' behavior recognition. The recognition rate of the algorithm was improved by 9. 9% after the processing of PCA, and the recognition effect was improved significantly. |
Key words: ewes house feeding prenatal behavior clustering algorithm neural network behavior recognition |