本文已被:浏览 611次 下载 349次 |
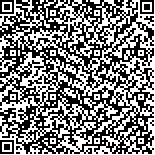 码上扫一扫! |
基于数码图像的甜菜氮素近地遥感监测模型 |
张珏1,2, 田海清1, 李哲1, 李斐3, 史树德4
|
|
(1.内蒙古农业大学 机电工程学院, 呼和浩特 010018;2.内蒙古师范大学 物理与电子信息学院, 呼和浩特 010022;3.内蒙古农业大学 草原与资源环境学院, 呼和浩特 010018;4.内蒙古农业大学 农学院, 呼和浩特 010018) |
|
摘要: |
为定量研究利用数码图像进行甜菜冠层叶片氮含量(Leaf nitrogen content,LNC)时空变化监测的适宜性及准确性,以2014年内蒙古赤峰市松山区太平地镇田间试验为基础,在甜菜各生长阶段采集甜菜冠层数码图像,利用数字图像处理技术对图像进行分割并提取红光值(R)、绿光值(G)和蓝光值(B)。分析R/B、G/B等9个颜色参数与不同生育期冠层LNC的相关性,并研究冠层LNC随施氮量的变化规律,探寻适宜于甜菜氮素营养监测的关键生育时期及最佳颜色参数。分别利用支持向量机(Support vector machine,SVM)和BP人工神经网络(Back-Propagation artificial neural network,BP-ANN)建立甜菜冠层LNC预测模型。研究结果表明,BP-ANN预测模型具有较高且较稳定的预测精度,其验证集的决定系数R2和均方根误差RMSE分别为0.74和2.35,与SVM模型相比,BP-ANN模型的决定系数R2提高了12.12%,均方根误差RMSE降低了8.09%。 |
关键词: 甜菜 氮素监测 颜色参数 叶片氮含量 数码图像 |
DOI:10.11841/j.issn.1007-4333.2018.06.15 |
投稿时间:2017-07-29 |
基金项目:国家自然科学基金项目(41261084);内蒙古自然科学基金项目(2016MS0346) |
|
Remote sensing model for monitoring nitrogen in beet based on digital imaging |
ZHANG Jue1,2, TIAN Haiqing1, LI Zhe1, LI Fei3, SHI Shude4
|
(1.College of Mechanical and Electrical Engineering, Inner Mongolia Agricultural University, Hohhot 010018, China;2.College of Physics and Electronic Information, Inner Mongolia Normal University, Hohhot 010022, China;3.College of Grassland, Resources and Environment, Inner Mongolia Agricultural University, Hohhot 010018, China;4.College of Agronomy, Inner Mongolia Agricultural University, Hohhot 010018, China) |
Abstract: |
To explore the feasibility of monitoring nitrogen elements in beet canopy leaves based on digital imaging, field experiments with different planting schemes were carried out in Chifeng City, Inner Mongolia on 2014.To examine the effects of N status on beet growth and color information, four N treatments ranging from 0 to 163 kg/hm2 were applied for growing season.Digital images of beet grown under different nitrogen application rates were taken for several times during the whole growth stage.Meanwhile, the beet plants were sampled to measure leaf nitrogen content (LNC).Digital image processing technology was used to extract image color information including the values of red, green, and blue light, and 9 color indices such as R/B, G/B were computed.In searching key growth stages and the best color parameters suitable for monitoring nitrogen nutrition of beet, correlation between the above color indices with beet LNC was analyzed, and the change regulation of canopy LNC with nitrogen application rate was studied.LNC prediction model of beet canopy was established using support vector machine(SVM)and back-propagation artificial neural network(BP-ANN)respectively.The results indicated that the BP-ANN model has higher and more stable prediction accuracy.The R2 and RMSE of verification set were 0.74 and 2.35, respectively.Compared with the SVM model, the R2 of BP-ANN increased by 12.12%, and RMSE was reduced by 8.09% respectively.Above results indicated that digital image processing technique could be used for the non-destructive diagnosis of crop nitrogen nutrition. |
Key words: beet nutrition monitoring color parameter leaf nitrogen concentration digital image |